Moneyball Was Just the Beginning; Big Data Analysis in Sport
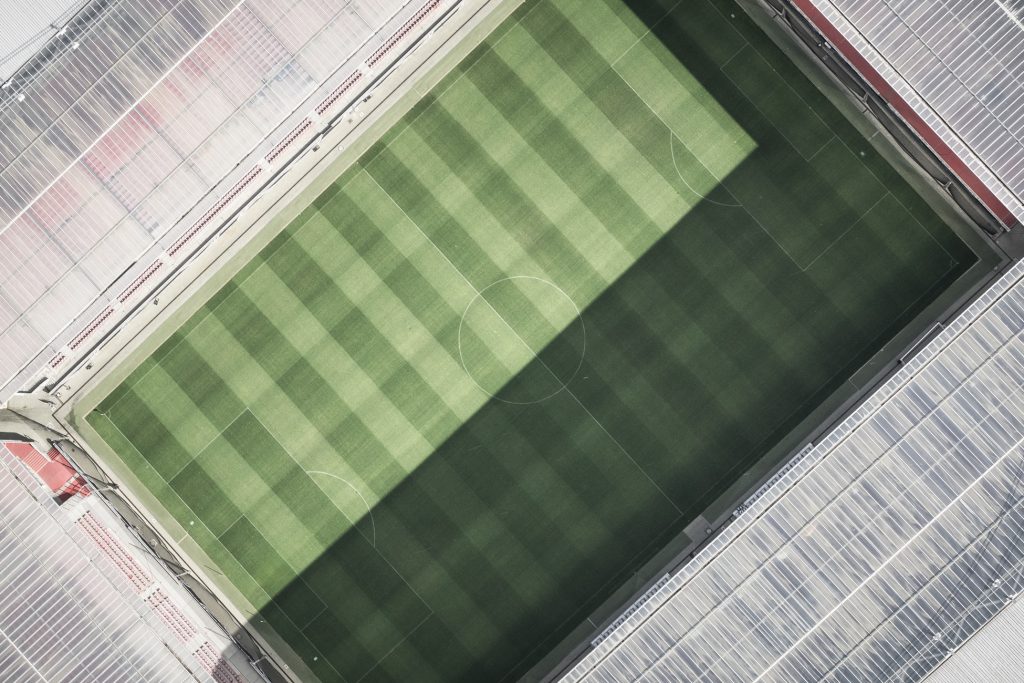
Most People are familiar with the story of Moneyball: in 2002 the Oakland Athletics, spearheaded by general manager Billy Beane, won more regular seasons games than every other team but the Atlanta Braves, in spite of having the second lowest payroll in the league. Beane realised that the A’s could never compete financially with the Yankees, Red Sox or Dodgers so he had to box smarter; look for ‘inefficiencies in the game’. He and his staff began to investigate undervalued skills such as ‘foot speed’ and ‘on base percentage’ and recruited free agents based on these metrics. After The A’s tremendously successful season of 2002, The Red Sox (having unsuccessfully tried to hire Billy Beane along the way) would adopt a similar approach and win their first World Series for 84 years.
Big data analytics has since exploded onto the sports scene. More and more sports teams around the world are turning to raw data sets to better evaluate and plan on and off the field of play. It has completely revolutionised the way some sports are played. With instant access to terabytes of data, teams can draw definitive conclusions in seconds, which would have previously required hours of examining footage. A clear sign at how far advanced this field is becoming – Massachusetts Institute of Technology are showing an interest. They run a yearly conference on the topic with its most distinguished minds proposing fresh ways to revolutionise sport using Big Data.
So how does it work? “Sports are watched by millions and millions of people – yet, pretty much all of the strategic decisions are made by humans in a split second. These decisions could definitely be enhanced by learning from past data, but humans can’t keep large databases in their heads” Cynthia Rudin, associate professor of statistics at MIT, told the Guardian in 2014. So in that split second, with everything else held constant, if that player is able to make a better informed decision his chances of being successful improve.
It all comes down to spotting a pattern in the data and interpreting whether this pattern adds value. The Leicester Tigers use IBM’s predictive analysis software to measure fatigue level and game intensity. This assesses injury risks and then delivers training programs for players at risk, thus helping them keep their best players on the pitch for the longer. However, using the data as a means of examining game play becomes more difficult. Baseball is a game that is driven by ‘black and white’ metrics, but games that are played in a continuous flow, e.g. Football, Rugby and Hockey, make the game more difficult to put into an analysis on paper.
Arsenal have invested millions of pounds recently to build its own analytics team to make better use of the data that is available to them. There are 8 cameras installed around the Emirates Stadium to track every player and their interactions. The sports analytics provider Prozone tracks 10 data points per player per second (c. 1.4 million data points a game) and these are analysed using automated algorithms as well as manual coding of every interaction with the ball to increase the accuracy and value of the analysis. The suggestions emerging are the most value is to be found in ‘off the ball’ figures. Players will only spend a fraction of each game directly interacting with the ball, and far more time getting into positions where they can affect the game – getting in dangerous positions or disrupting the flow of the opposing team. This is where a lot of untapped potential can be found in the data. Kevin Mongeon, the principal owner at The Sports Analytics Institute believes one day that statistical models will be developed that can examine a player’s abilities under different scenarios, but we are still short of having the data that gets us to this point.
In NFL, the analysis of almost 12,000 field goal attempts has conclusively returned results that show environmental factors, such as wind velocity and temperature, are the leading metric that determines success ratio. It may sound obvious on the face of it, but for years it was assumed that psychological factors were the reason behind failed goaled attempts, which often meant calling a timeout to ice a kicker and allow him a chance to compose himself, when perhaps this time could have been better spent.
This is not the only myth that has been categorically debunked by the analysis of big data. In Paul Tomkins brilliant book, Pay as you Play, Tomkins crunches data from over 20 years of Premier League transfers. One of the more interesting conclusions is a complete rejection of the notion of ‘Premier League experience’ in a transfer being a positive factor. It’s neither a good metric nor a bad one, simply irrelevant. He also concludes that only 40% of transfers can be deemed successful. Perhaps some further analysis could help isolate a combination of metrics that lead to a successful transfer, to avoid further high profile blunders.
Will the future advances in big data analysis benefit sport? Unquestionable benefits will come from areas such as GPS tracking, Omegawave analysis, brain-wave analysis and RestQ-52 to evaluate players’ health. While this keeps the average player fitter and healthier, it should also help prevent tragic on-field incidences, most recently epitomised by Gregory Mertens of Belgium collapsing and dying on the pitch with very little warning.
Coaches stand to benefit too. During an NFL game, for example, coaches in the dugout will have access to complex data algorithms that will leverage opponent’s tendencies with the current game situation and provide suggestions of how to exploit them in real time.
So why hasn’t big data analysis been rolled out industry wide in sports like Football? There is still a feeling among the professionals in the game that the “sports guys know more than the numbers guys”. They point to examples of the stats guys getting it wrong, such as Damien Comolli’s ill-fated spell at Liverpool for reasons why it simply can’t work in a game as nuanced and unpredictable as Football. Perhaps it bourns from a fear that, if it does work, there will be no place left for them in the game.
Perhaps a fitting ending for this blog, as this is a fear that is not unique to sport. Stephen Cohen, co-founder of the big data analysis startup Palantir, delivered a presentation at Wired 2012 aimed at dispelling these fears. No matter how far advanced the analysis becomes, “Humans are fundamentally of a greater order than algorithms”. The data can only tell you so much, so when the human being is needed to interpret that data, there will be no one more qualified than the sports guys to provide that service.
Oxford Knight is a technical recruitment agency. None of our consultants have written a line of code... yet. We apologise if this article doesn’t keep some purist happy, but we’re trying to build a new generation of technical recruitment agencies…. We listen, participate, and deliver.